Note
Go to the end to download the full example code
Optimal Transport in 2D
Let’s use the gradient of the Sinkhorn divergence to compute an Optimal Transport map.
Setup
import numpy as np
import matplotlib.pyplot as plt
import time
import torch
from geomloss import SamplesLoss
use_cuda = torch.cuda.is_available()
dtype = torch.cuda.FloatTensor if use_cuda else torch.FloatTensor
Display routines
from random import choices
from imageio import imread
def load_image(fname):
img = imread(fname, mode="F") # Grayscale
img = (img[::-1, :]) / 255.0
return 1 - img
def draw_samples(fname, n, dtype=torch.FloatTensor):
A = load_image(fname)
xg, yg = np.meshgrid(
np.linspace(0, 1, A.shape[0]),
np.linspace(0, 1, A.shape[1]),
indexing="xy",
)
grid = list(zip(xg.ravel(), yg.ravel()))
dens = A.ravel() / A.sum()
dots = np.array(choices(grid, dens, k=n))
dots += (0.5 / A.shape[0]) * np.random.standard_normal(dots.shape)
return torch.from_numpy(dots).type(dtype)
def display_samples(ax, x, color):
x_ = x.detach().cpu().numpy()
ax.scatter(x_[:, 0], x_[:, 1], 25 * 500 / len(x_), color, edgecolors="none")
Dataset
Our source and target samples are drawn from measures whose densities are stored in simple PNG files. They allow us to define a pair of discrete probability measures:
N, M = (100, 100) if not use_cuda else (10000, 10000)
X_i = draw_samples("data/density_a.png", N, dtype)
Y_j = draw_samples("data/density_b.png", M, dtype)
/home/code/geomloss/geomloss/examples/optimal_transport/plot_optimal_transport_2D.py:33: DeprecationWarning: Starting with ImageIO v3 the behavior of this function will switch to that of iio.v3.imread. To keep the current behavior (and make this warning disappear) use `import imageio.v2 as imageio` or call `imageio.v2.imread` directly.
img = imread(fname, mode="F") # Grayscale
Lagrangian gradient descent
def gradient_descent(loss, lr=1):
"""Flows along the gradient of the loss function.
Parameters:
loss ((x_i,y_j) -> torch float number):
Real-valued loss function.
lr (float, default = 1):
Learning rate, i.e. time step.
"""
# Parameters for the gradient descent
Nsteps = 11
display_its = [0, 1, 2, 10]
# Use colors to identify the particles
colors = (10 * X_i[:, 0]).cos() * (10 * X_i[:, 1]).cos()
colors = colors.detach().cpu().numpy()
# Make sure that we won't modify the reference samples
x_i, y_j = X_i.clone(), Y_j.clone()
# We're going to perform gradient descent on Loss(α, β)
# wrt. the positions x_i of the diracs masses that make up α:
x_i.requires_grad = True
t_0 = time.time()
plt.figure(figsize=(12, 12))
k = 1
for i in range(Nsteps): # Euler scheme ===============
# Compute cost and gradient
L_αβ = loss(x_i, y_j)
[g] = torch.autograd.grad(L_αβ, [x_i])
if i in display_its: # display
ax = plt.subplot(2, 2, k)
k = k + 1
plt.set_cmap("hsv")
plt.scatter(
[10], [10]
) # shameless hack to prevent a slight change of axis...
display_samples(ax, y_j, [(0.55, 0.55, 0.95)])
display_samples(ax, x_i, colors)
ax.set_title("it = {}".format(i))
plt.axis([0, 1, 0, 1])
plt.gca().set_aspect("equal", adjustable="box")
plt.xticks([], [])
plt.yticks([], [])
plt.tight_layout()
# in-place modification of the tensor's values
x_i.data -= lr * len(x_i) * g
plt.title(
"it = {}, elapsed time: {:.2f}s/it".format(i, (time.time() - t_0) / Nsteps)
)
Wasserstein-2 Optimal Transport
Sinkhorn divergences rely on blurry transport plans
However, when p = 2, we can interpret the gradient field
gradient_descent(SamplesLoss("sinkhorn", p=2, blur=0.1))
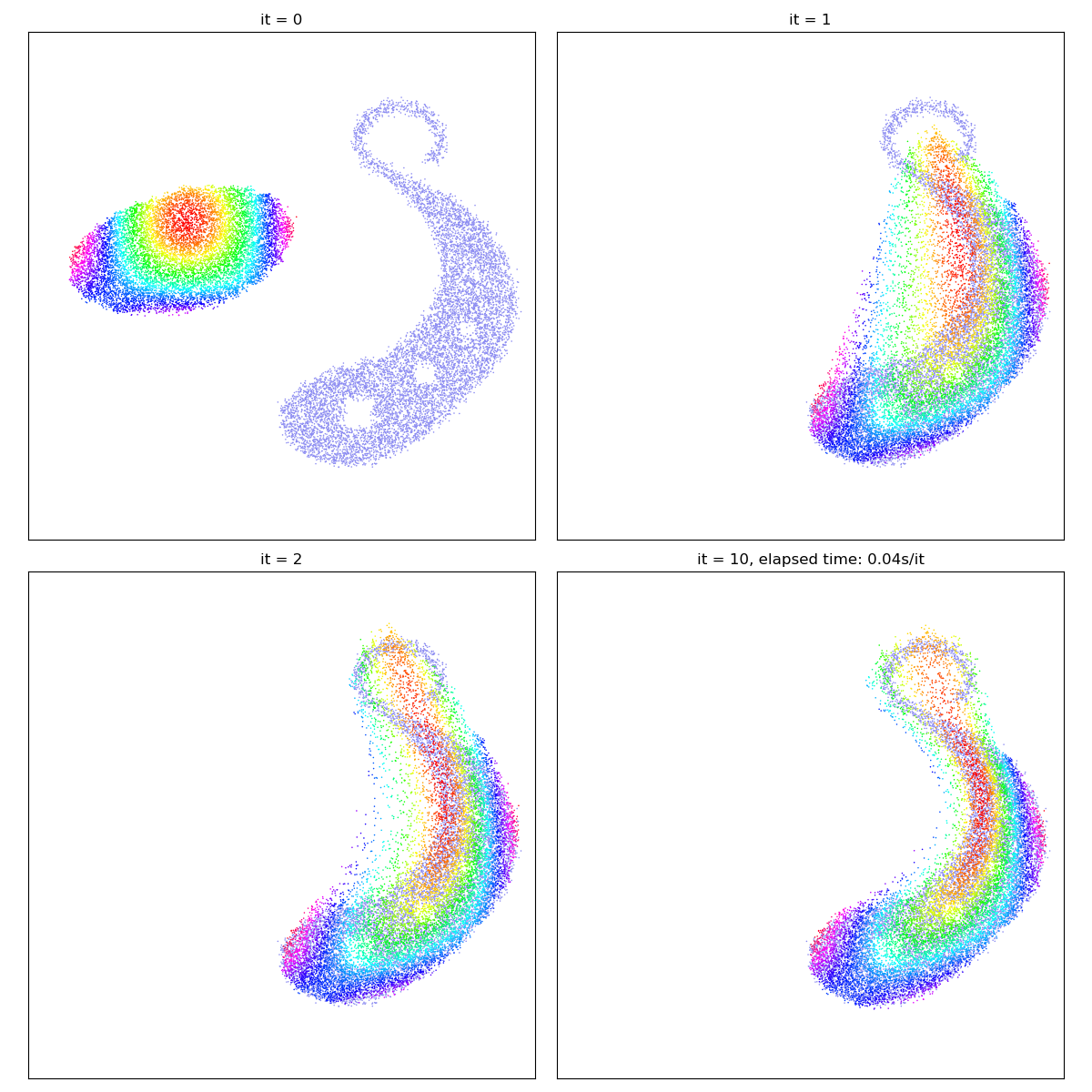
Crucially, as the blurring scale
gradient_descent(SamplesLoss("sinkhorn", p=2, blur=0.01))
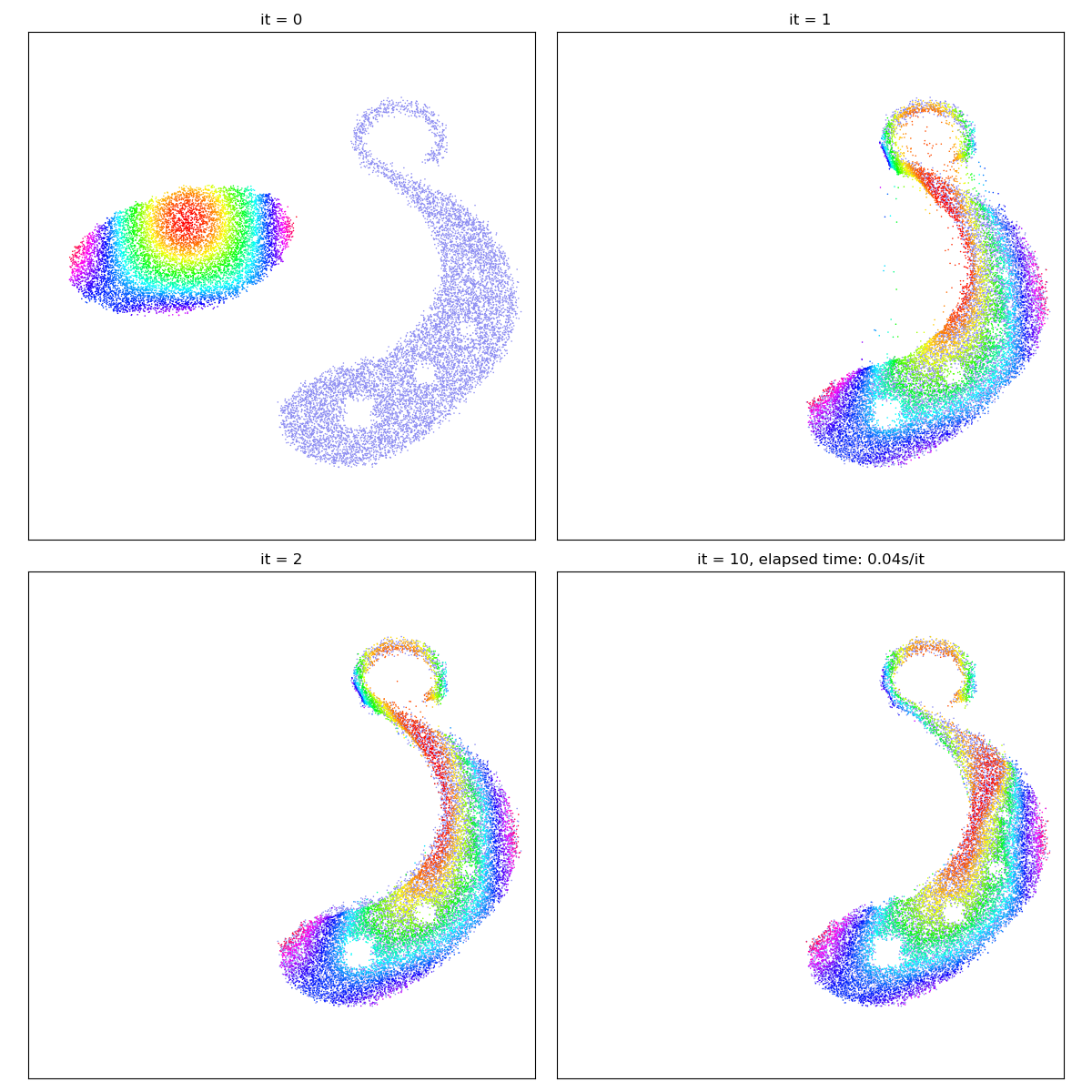
The reach
parameter allows us to introduce laziness
into the classical Monge problem, specifying a maximum
scale (half-life) of interaction between the
gradient_descent(SamplesLoss("sinkhorn", p=2, blur=0.01, reach=0.1))
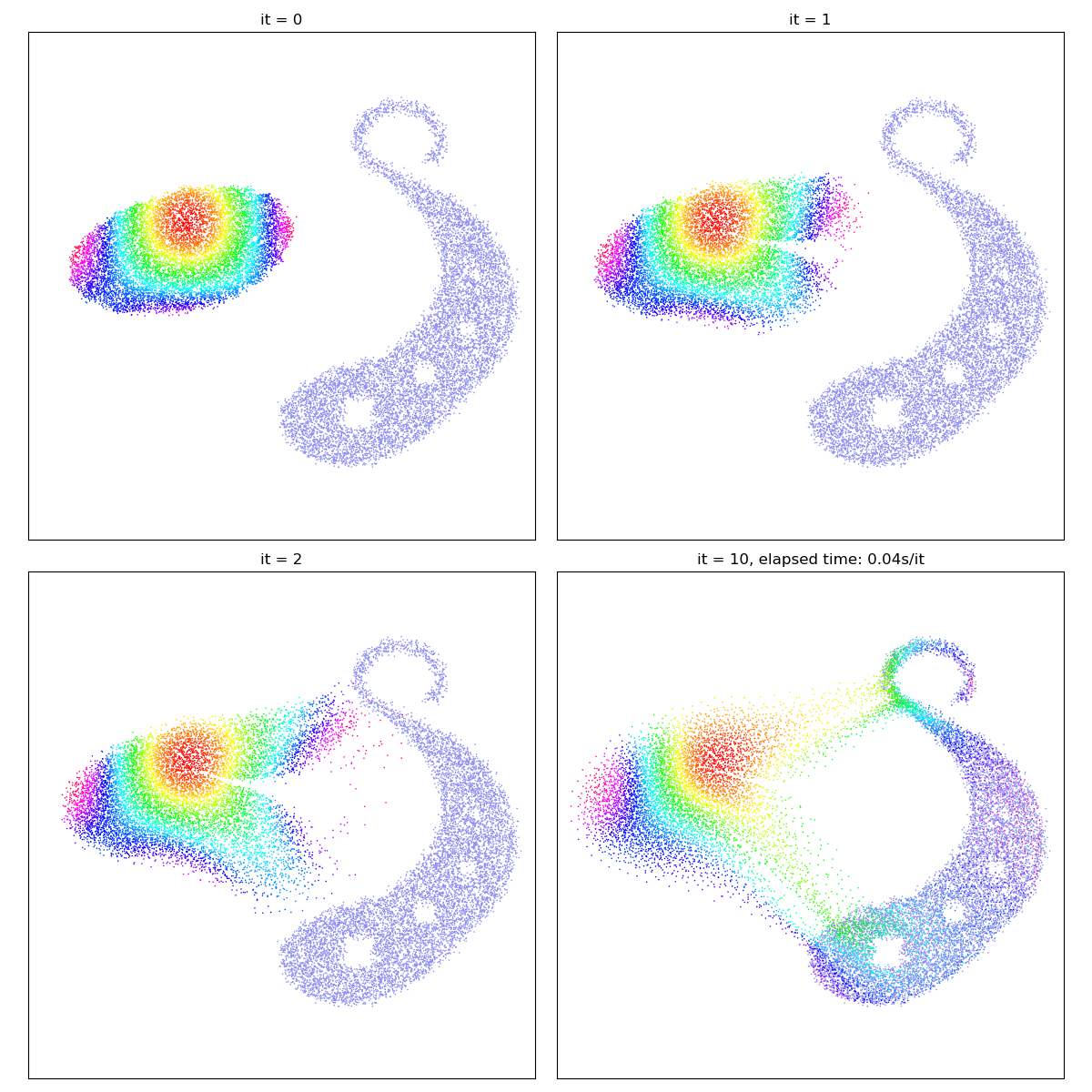
Optimal Transport is not the panacea
Optimal Transport theory is all about discarding the topological structure of the data to get a simple, convex registration algorithm: the Monge map transports bags of sands from one location to another, and may tear shapes apart as needed.
In generative modelling, this versatility allows us to fit “Gaussian blobs” to any kind of empirical distribution:
X_i = draw_samples("data/crescent_a.png", N, dtype)
Y_j = draw_samples("data/crescent_b.png", M, dtype)
gradient_descent(SamplesLoss("sinkhorn", p=2, blur=0.01))
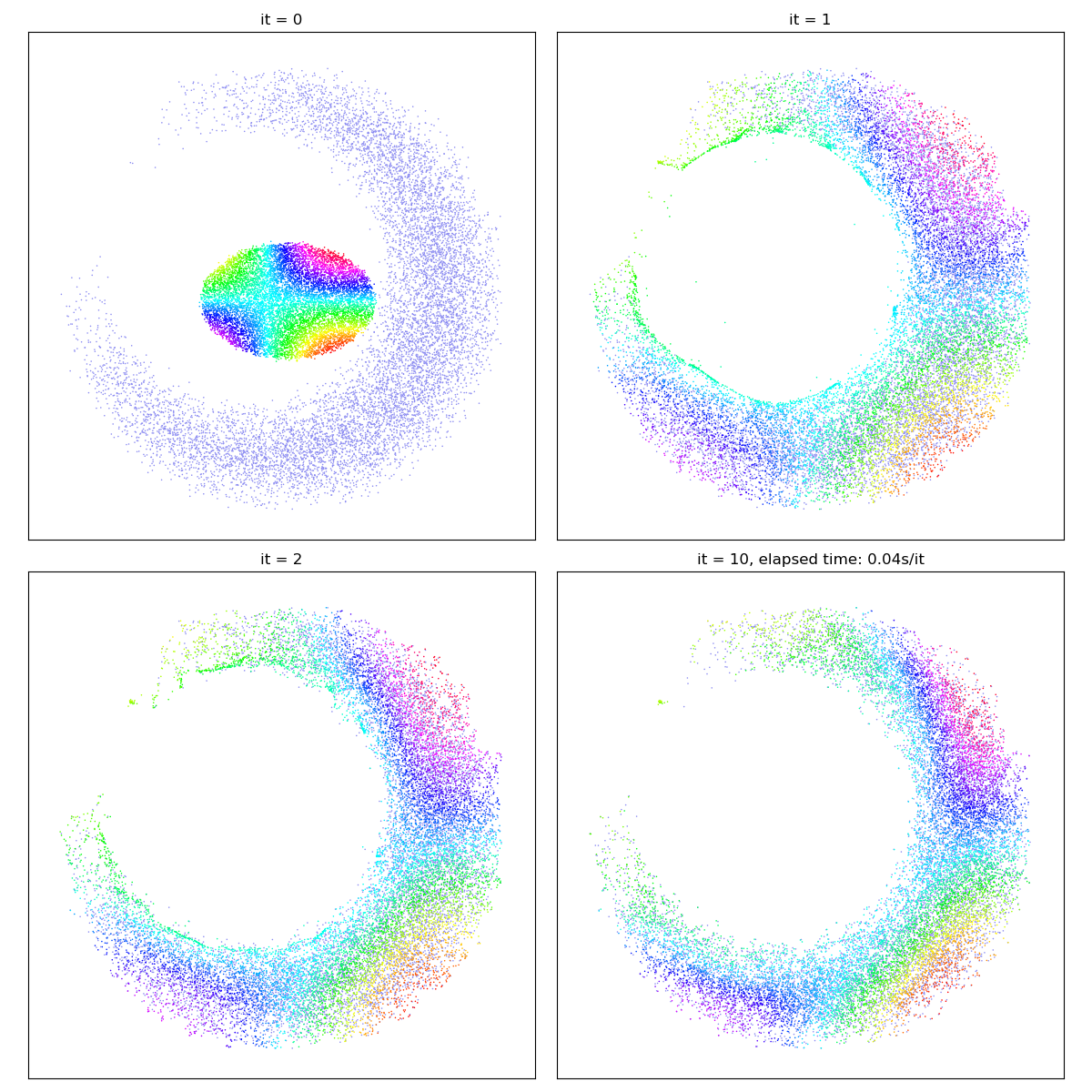
/home/code/geomloss/geomloss/examples/optimal_transport/plot_optimal_transport_2D.py:33: DeprecationWarning: Starting with ImageIO v3 the behavior of this function will switch to that of iio.v3.imread. To keep the current behavior (and make this warning disappear) use `import imageio.v2 as imageio` or call `imageio.v2.imread` directly.
img = imread(fname, mode="F") # Grayscale
Going further, in simple situations, Optimal Transport may even be used as a “cheap and easy” registration routine…
X_i = draw_samples("data/worm_a.png", N, dtype)
Y_j = draw_samples("data/worm_b.png", M, dtype)
gradient_descent(SamplesLoss("sinkhorn", p=2, blur=0.01))
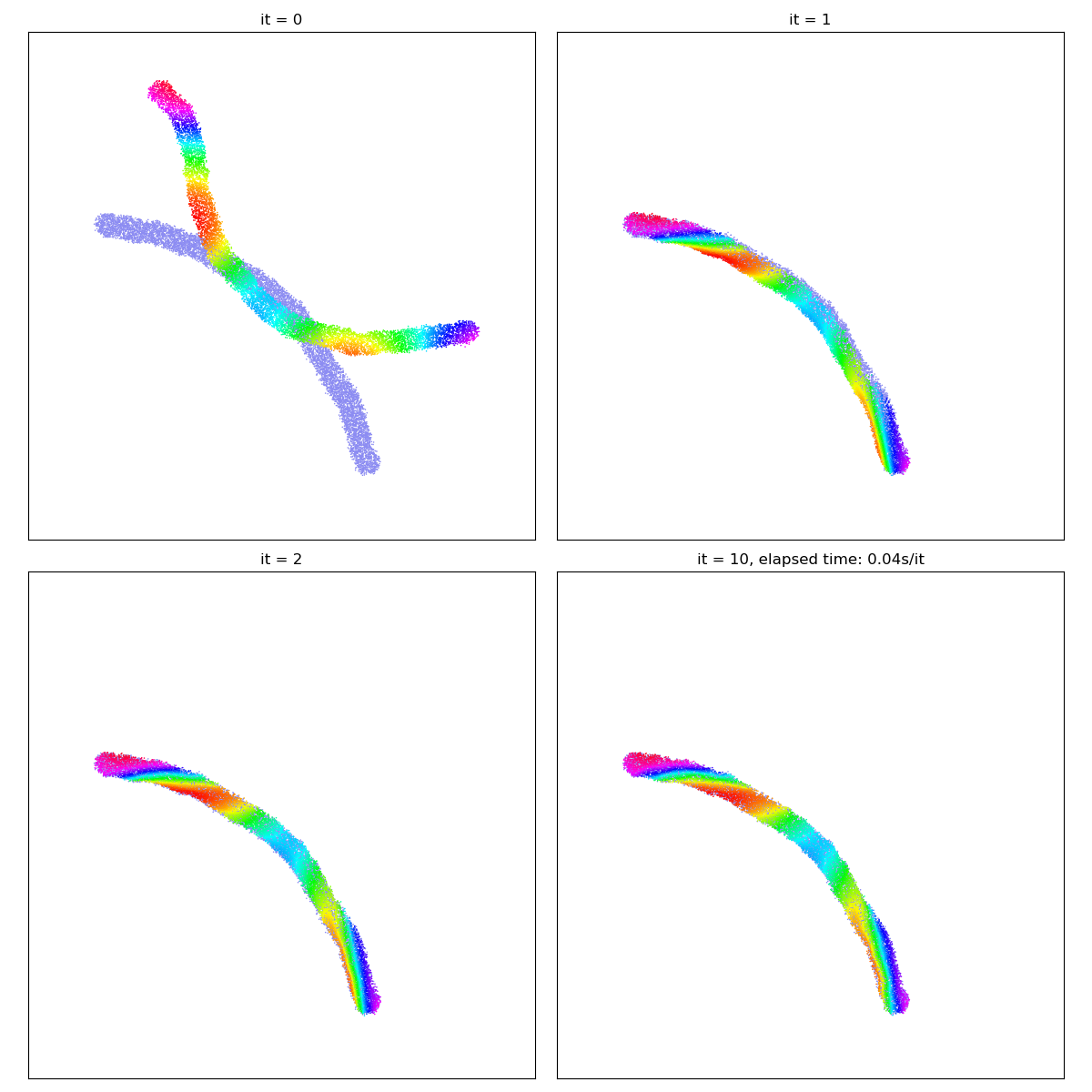
/home/code/geomloss/geomloss/examples/optimal_transport/plot_optimal_transport_2D.py:33: DeprecationWarning: Starting with ImageIO v3 the behavior of this function will switch to that of iio.v3.imread. To keep the current behavior (and make this warning disappear) use `import imageio.v2 as imageio` or call `imageio.v2.imread` directly.
img = imread(fname, mode="F") # Grayscale
But beware! Out-of-the-box, Optimal Transport will not match the salient features of both shapes (e.g. ends or corners) with each other. In real-life applications, Sinkhorn divergences should thus always be used in a relevant feature space (e.g. of SIFT descriptors), in conjunction with a prior-enforcing generative model (e.g. a convolutional neural network or a thin plate spline deformation).
X_i = draw_samples("data/moon_a.png", N, dtype)
Y_j = draw_samples("data/moon_b.png", M, dtype)
gradient_descent(SamplesLoss("sinkhorn", p=2, blur=0.01))
plt.show()
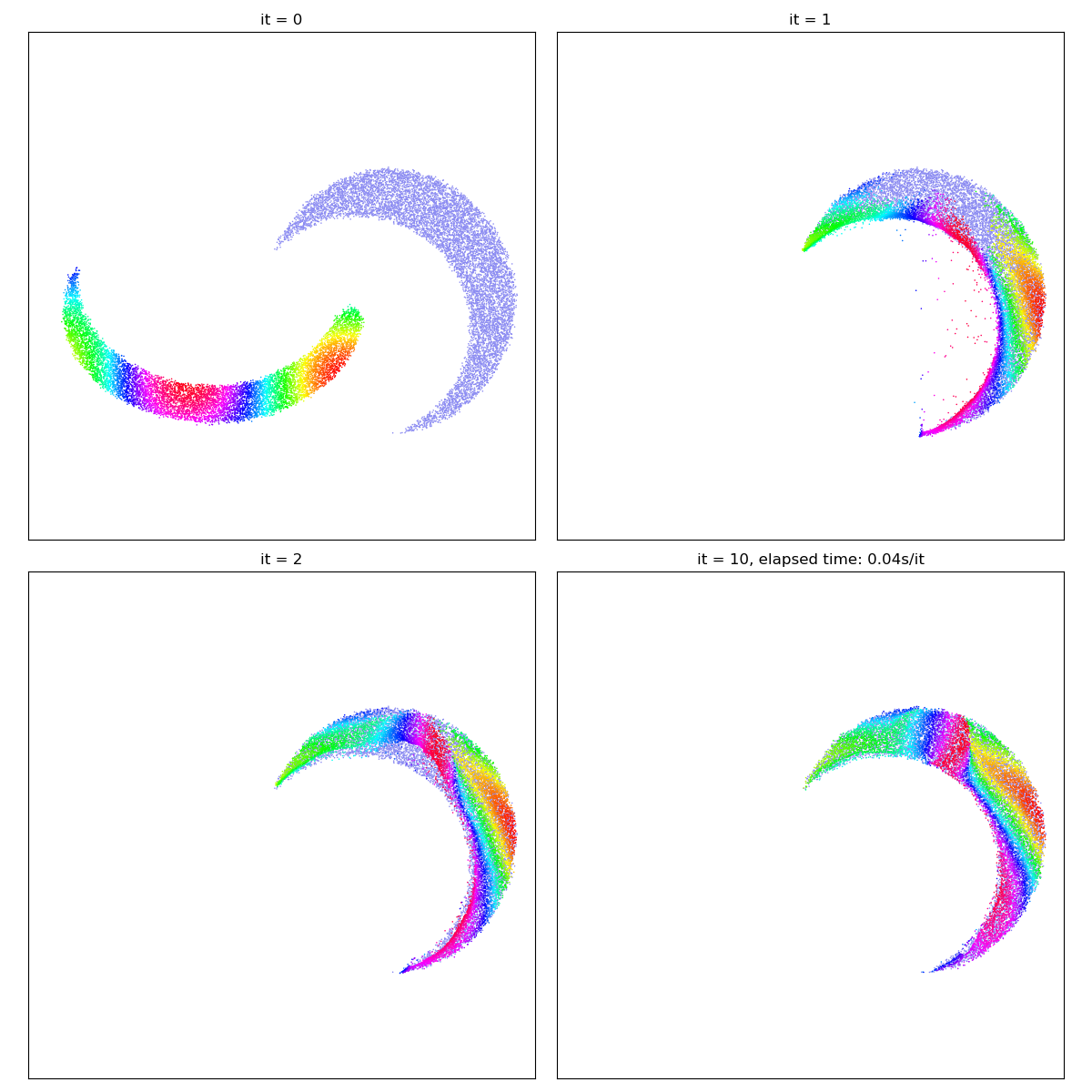
/home/code/geomloss/geomloss/examples/optimal_transport/plot_optimal_transport_2D.py:33: DeprecationWarning: Starting with ImageIO v3 the behavior of this function will switch to that of iio.v3.imread. To keep the current behavior (and make this warning disappear) use `import imageio.v2 as imageio` or call `imageio.v2.imread` directly.
img = imread(fname, mode="F") # Grayscale
Total running time of the script: (0 minutes 4.490 seconds)