Note
Go to the end to download the full example code
Color transfer with Optimal Transport
Let’s use the gradient of the Sinkhorn divergence to change the color palette of an image.
Setup
import numpy as np
import matplotlib.pyplot as plt
from mpl_toolkits.mplot3d import Axes3D
import time
import torch
from geomloss import SamplesLoss
use_cuda = torch.cuda.is_available()
dtype = torch.cuda.FloatTensor if use_cuda else torch.FloatTensor
Display routines
import numpy as np
import torch
from random import choices
import imageio
from matplotlib import pyplot as plt
def load_image(fname):
img = imageio.imread(fname) # RGB
return img / 255.0 # Normalized to [0,1]
def RGB_cloud(fname, sampling, dtype=torch.FloatTensor):
A = load_image(fname)
A = A[::sampling, ::sampling, :]
return torch.from_numpy(A).type(dtype).view(-1, 3)
def display_cloud(ax, x):
x_ = x.detach().cpu().numpy()
ax.scatter(x_[:, 0], x_[:, 1], x_[:, 2], s=25 * 500 / len(x_), c=x_)
def display_image(ax, x):
W = int(np.sqrt(len(x)))
x_ = x.view(W, W, 3).detach().cpu().numpy()
ax.imshow(x_)
Dataset
Our source and target samples are clouds of 3D points,
each of whom encodes the RGB color of a pixel
in a standard test image. We can then define a pair of discrete
probability measures on our color space
sampling = 8 if not use_cuda else 1
X_i = RGB_cloud("data/house_256.png", sampling, dtype)
Y_j = RGB_cloud("data/mandrill_256.png", sampling, dtype)
fig = plt.figure(figsize=(12, 12))
ax = fig.add_subplot(2, 2, 1)
display_image(ax, X_i)
ax.set_title("Source image")
ax = fig.add_subplot(2, 2, 2)
display_image(ax, Y_j)
ax.set_title("Target image")
ax = fig.add_subplot(2, 2, 3, projection="3d")
display_cloud(ax, X_i)
ax.set_title("Source point cloud")
ax = fig.add_subplot(2, 2, 4, projection="3d")
display_cloud(ax, Y_j)
ax.set_title("Target point cloud")
plt.tight_layout()
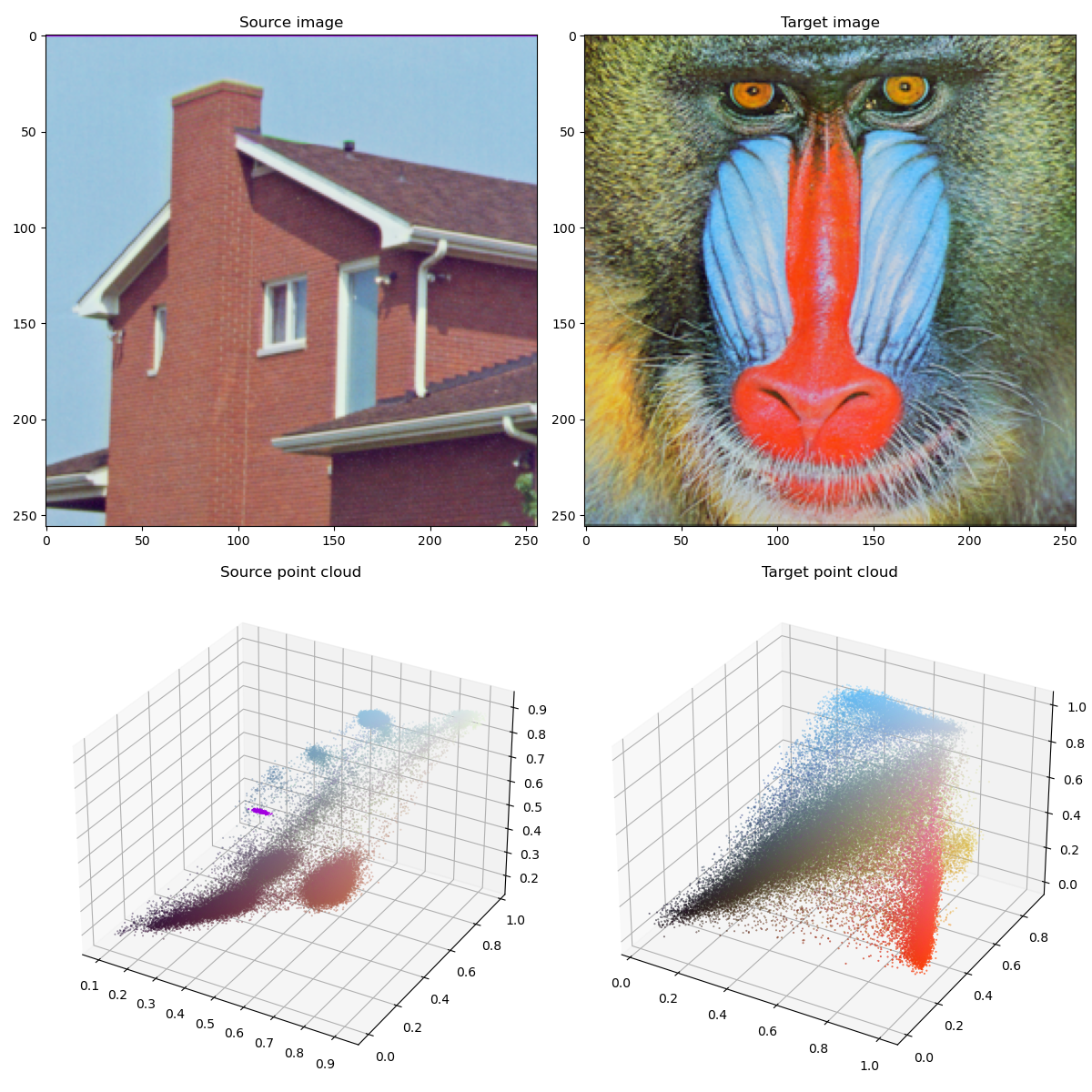
/home/code/geomloss/geomloss/examples/optimal_transport/plot_optimal_transport_color.py:38: DeprecationWarning: Starting with ImageIO v3 the behavior of this function will switch to that of iio.v3.imread. To keep the current behavior (and make this warning disappear) use `import imageio.v2 as imageio` or call `imageio.v2.imread` directly.
img = imageio.imread(fname) # RGB
Color transfer through gradient descent
To showcase the properties of the Sinkhorn divergence
def color_transfer(loss, lr=1):
"""Flows along the gradient of the loss function.
Parameters:
loss ((x_i,y_j) -> torch float number):
Real-valued loss function.
lr (float, default = 1):
Learning rate, i.e. time step.
"""
# Parameters for the gradient descent
Nsteps = 11
display_its = [1, 10]
# Make sure that we won't modify the reference samples
x_i, y_j = X_i.clone(), Y_j.clone()
# We're going to perform gradient descent on Loss(α, β)
# wrt. the positions x_i of the diracs masses that make up α:
x_i.requires_grad = True
t_0 = time.time()
plt.figure(figsize=(12, 12))
k = 3
ax = plt.subplot(2, 2, 1)
display_image(ax, X_i)
ax.set_title("Source image")
plt.xticks([], [])
plt.yticks([], [])
ax = plt.subplot(2, 2, 2)
display_image(ax, Y_j)
ax.set_title("Target image")
plt.xticks([], [])
plt.yticks([], [])
for i in range(Nsteps): # Euler scheme ===============
# Compute cost and gradient
L_αβ = loss(x_i, y_j)
[g] = torch.autograd.grad(L_αβ, [x_i])
if i in display_its: # display
ax = plt.subplot(2, 2, k)
display_image(ax, x_i)
ax.set_title("it = {}".format(i))
k = k + 1
plt.xticks([], [])
plt.yticks([], [])
# in-place modification of the tensor's values
x_i.data -= lr * len(x_i) * g
plt.title(
"it = {}, elapsed time: {:.2f}s/it".format(i, (time.time() - t_0) / Nsteps)
)
plt.tight_layout()
Wasserstein-2 Optimal Transport
When p = 2, the (normalized) Lagrangian gradient of the Sinkhorn divergence
Crucially, when
color_transfer(SamplesLoss("sinkhorn", blur=0.3))
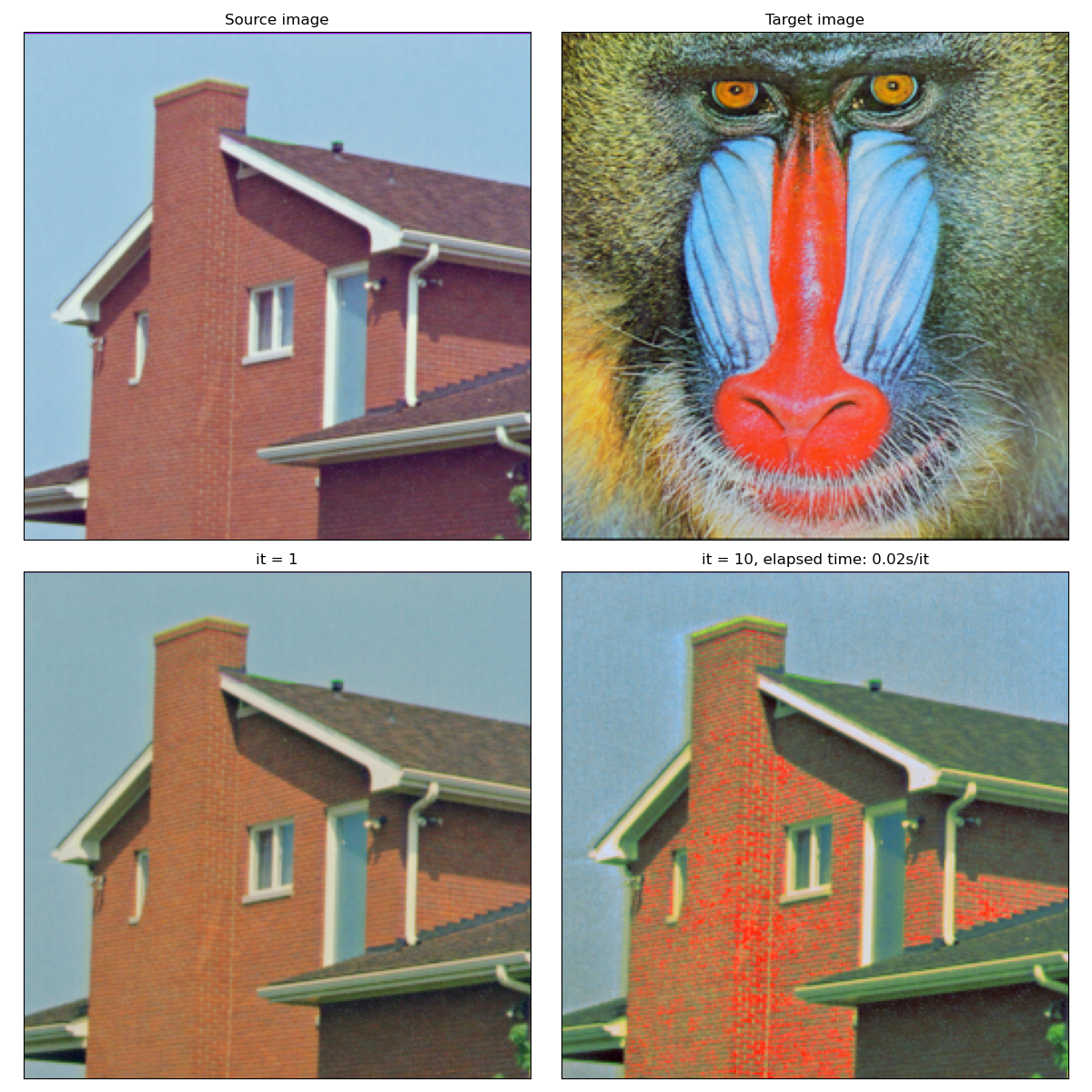
Clipping input data to the valid range for imshow with RGB data ([0..1] for floats or [0..255] for integers). Got range [-9.75281e-06..0.88715476].
Clipping input data to the valid range for imshow with RGB data ([0..1] for floats or [0..255] for integers). Got range [-0.023618542..1.2329485].
In most applications, the color transfer obtained after one, smooth update is more appropriate than the “perfect” matching, solution of the Monge problem. Fortunately, this smooth color transfer is also easier to compute!
Feel free to play around with the input features (i.e. the coordinates system on the color space) and the blur parameter, which allows you to be more or less precise in the first few iterations:
color_transfer(SamplesLoss("sinkhorn", blur=0.1))
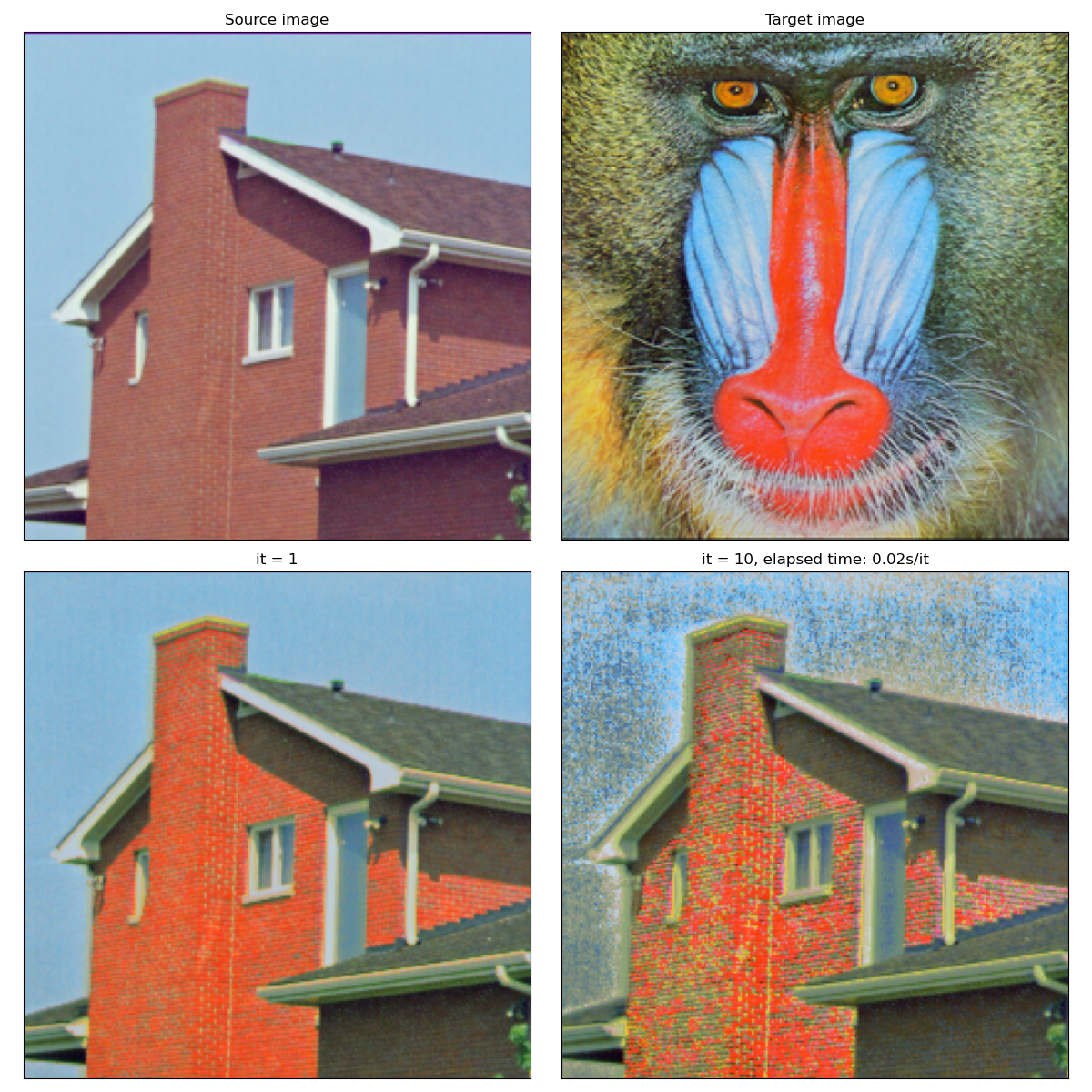
Clipping input data to the valid range for imshow with RGB data ([0..1] for floats or [0..255] for integers). Got range [0.012572595..1.0214131].
Clipping input data to the valid range for imshow with RGB data ([0..1] for floats or [0..255] for integers). Got range [-0.015074465..1.0201979].
Going further, the reach parameter allows you to define a maximum transportation distance in the color space. In real-life applications, you may want to apply this simple algorithm on a higher-dimensional feature space (e.g. position + color), and thus get quasi-smooth matchings at a low computational cost.
color_transfer(SamplesLoss("sinkhorn", blur=0.1, reach=0.4))
plt.show()
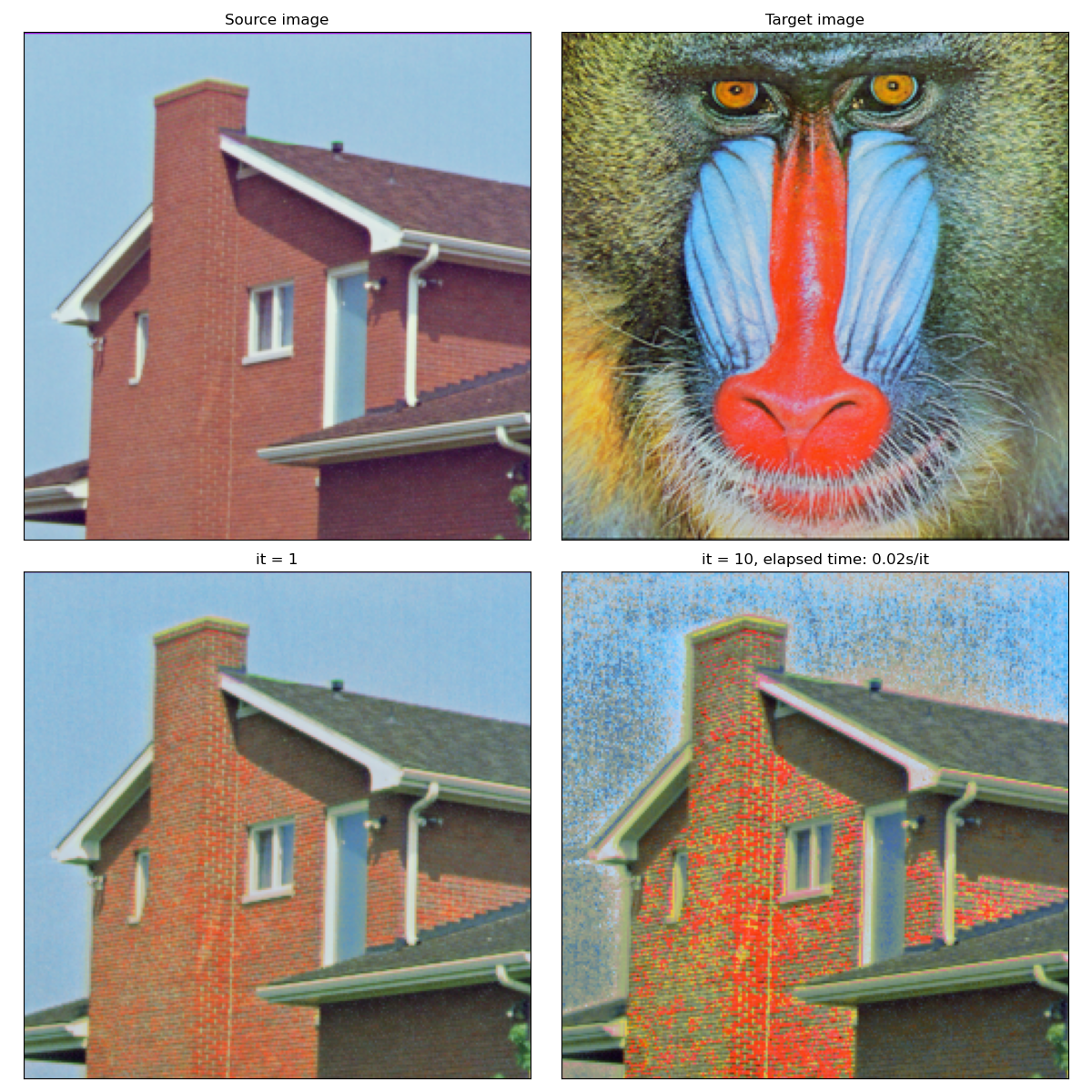
Clipping input data to the valid range for imshow with RGB data ([0..1] for floats or [0..255] for integers). Got range [-0.017584927..1.0228894].
Total running time of the script: (0 minutes 5.285 seconds)